In the digital age, the quality of video content is paramount. As we navigate through an era where visual content is king, the importance of video quality metrics cannot be overstated. These metrics serve as the yardstick by which we measure the quality of video content, and they play a crucial role in shaping the viewer's experience. Among the tools that we use to assess video quality, AVQT, VMAF, and SSIMPLUS stand out. These tools provide us with a comprehensive understanding of video quality, allowing us to optimize our content for the best viewer experience. In this article, we delve into the world of video quality metrics, exploring the intricacies of AVQT, VMAF, and SSIMPLUS.
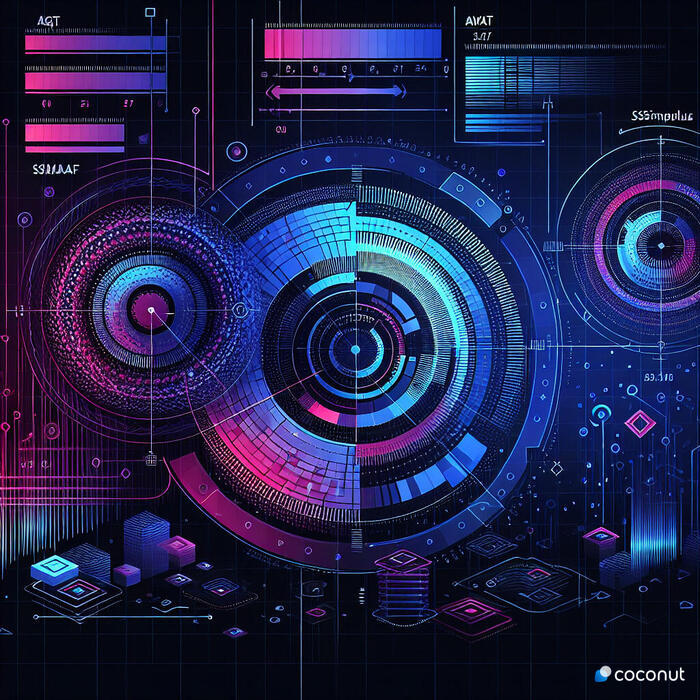
Demystifying Video Quality Metrics
Decoding Video Quality Metrics
Video quality metrics are essentially tools that we use to measure the quality of video content. They provide us with a quantitative assessment of the video's visual quality, allowing us to understand how well the video is likely to be perceived by the viewer. These metrics take into account various factors such as resolution, bitrate, frame rate, and compression artifacts, among others. The higher the video quality metric, the better the video quality. By understanding these metrics, we can optimize our video content to provide the best possible viewing experience. AVQT, VMAF, and SSIMPLUS are among the most widely used video quality metrics, each with its unique approach to assessing video quality.
The Journey of Video Quality Assessment
The evolution of video quality assessment has been a fascinating journey. In the early days, video quality was assessed primarily based on subjective evaluations. Viewers would watch a video and rate its quality based on their personal perception. However, this approach was fraught with inconsistencies and biases. As technology advanced, we began to develop objective metrics that could quantitatively assess video quality. These metrics provided a more reliable and consistent measure of video quality, paving the way for the development of sophisticated video quality assessment tools like AVQT, VMAF, and SSIMPLUS. Today, these tools form the backbone of our video quality assessment efforts, enabling us to deliver high-quality video content that meets the expectations of our viewers.
AVQT, VMAF, SSIMPLUS: The Titans of Video Quality Metrics
AVQT, VMAF, and SSIMPLUS are among the most widely used video quality metrics today. AVQT, or Audio Visual Quality Tool, is a tool that assesses both audio and video quality. It provides a comprehensive measure of the overall quality of the multimedia content. VMAF, or Video Multi-Method Assessment Fusion, is a metric developed by Netflix to assess video quality. It combines several existing metrics to provide a more accurate measure of perceived video quality. SSIMPLUS, on the other hand, is a tool that measures the quality of video content as perceived by the human visual system. It takes into account factors such as viewing conditions and screen resolution, providing a highly accurate measure of perceived video quality. By understanding these metrics and how they work, we can optimize our video content to provide the best possible viewing experience.
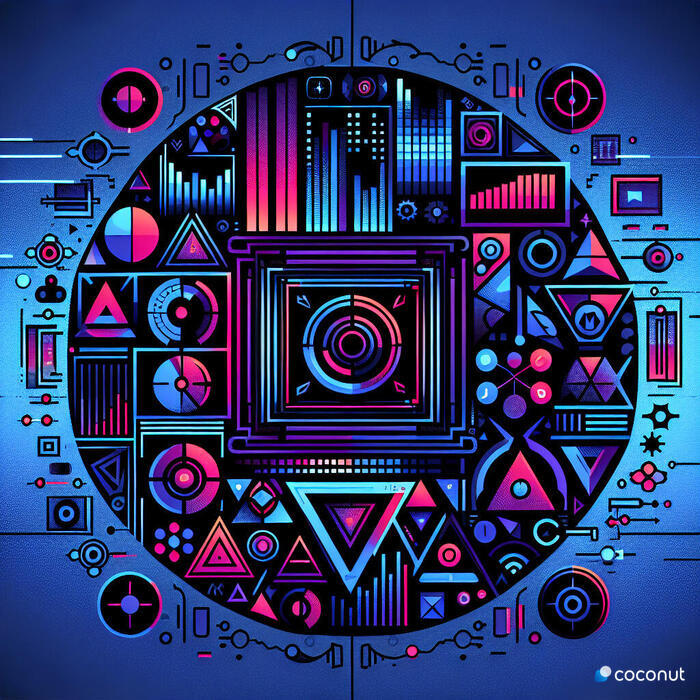
Historical Perspective on Video Quality Assessment and the Development of Various Metrics Over Time
The evolution of video quality assessment has been a fascinating journey, marked by continuous innovation and technological advancements. In the early days, the primary focus was on the physical aspects of video quality, such as resolution and frame rate. However, as technology advanced, the focus shifted towards more subjective aspects, such as the viewer's perception of quality. This led to the development of various metrics to measure video quality, each with its unique approach and methodology.The first generation of video quality metrics, such as Peak Signal-to-Noise Ratio (PSNR) and Mean Squared Error (MSE), were simple and easy to compute. However, they lacked the ability to accurately reflect the viewer's perception of quality. This led to the development of the second generation of metrics, such as the Structural Similarity Index (SSIM), which considered structural information in the video to provide a more accurate measure of perceived quality.The third generation of metrics, such as the Video Quality Metric (VQM) and the Moving Picture Quality Metric (MPQM), took a more holistic approach, considering multiple aspects of video quality, including spatial and temporal information, color, and contrast. These metrics provided a more comprehensive measure of video quality, but they were computationally intensive and required a reference video for comparison.The latest generation of metrics, such as the Advanced Video Quality Tool (AVQT), the Video Multimethod Assessment Fusion (VMAF), and SSIMPLUS, have taken video quality assessment to a new level. These metrics leverage machine learning and advanced algorithms to provide a highly accurate measure of perceived quality, without the need for a reference video. They also consider a wide range of factors, including viewing conditions and device capabilities, to provide a more personalized measure of video quality.
The Importance of Objective vs. Subjective Analysis
When it comes to video quality assessment, both objective and subjective analyses play crucial roles. Objective analysis involves the use of mathematical models and algorithms to quantify video quality. It provides a consistent and repeatable measure of quality, which is essential for benchmarking and comparison purposes. However, objective analysis may not always accurately reflect the viewer's perception of quality, as it does not consider subjective factors such as viewing conditions and personal preferences.On the other hand, subjective analysis involves the use of human observers to rate video quality. It provides a more accurate measure of perceived quality, as it considers subjective factors. However, subjective analysis is time-consuming, expensive, and may not be consistent or repeatable, as it is influenced by individual biases and variations in viewing conditions.Therefore, a comprehensive video quality assessment should involve both objective and subjective analyses. Objective analysis provides a consistent and repeatable measure of quality, while subjective analysis provides a more accurate measure of perceived quality. By combining the strengths of both methods, we can achieve a more comprehensive and accurate measure of video quality.
Deep Dive into AVQT, VMAF, and SSIMPLUS
AVQT: Advanced Video Quality Tool
The Advanced Video Quality Tool (AVQT) is a state-of-the-art video quality metric that leverages machine learning and advanced algorithms to provide a highly accurate measure of perceived quality. Unlike traditional metrics, AVQT does not require a reference video for comparison, making it more flexible and practical for real-world applications.AVQT considers a wide range of factors in its quality assessment, including spatial and temporal information, color, and contrast. It also considers viewing conditions and device capabilities, providing a more personalized measure of video quality. This makes AVQT highly effective for assessing the quality of video content delivered over the internet, where viewing conditions and device capabilities can vary widely.In the industry, AVQT is widely used for quality control, benchmarking, and optimization purposes. It provides a consistent and repeatable measure of quality, which is essential for comparing different video codecs, streaming protocols, and network conditions. It also provides valuable insights into the viewer's perception of quality, which can be used to optimize video encoding and delivery strategies.

VMAF: Video Multimethod Assessment Fusion
The Video Multimethod Assessment Fusion (VMAF) is a video quality metric developed by Netflix to measure the perceived quality of video content delivered over the internet. VMAF combines multiple quality metrics, including PSNR, SSIM, and Visual Information Fidelity (VIF), to provide a more comprehensive measure of quality.VMAF leverages machine learning to weight each metric based on its relevance to the viewer's perception of quality. This makes VMAF highly effective for assessing the quality of video content delivered over the internet, where viewing conditions and device capabilities can vary widely.In the industry, VMAF has become a standard for video quality measurement. It is widely used for quality control, benchmarking, and optimization purposes. It provides a consistent and repeatable measure of quality, which is essential for comparing different video codecs, streaming protocols, and network conditions. It also provides valuable insights into the viewer's perception of quality, which can be used to optimize video encoding and delivery strategies.
SSIMPLUS: A Step Beyond SSIM
SSIMPLUS is an advanced video quality metric that builds upon the strengths of the traditional SSIM metric. Like SSIM, SSIMPLUS considers structural information in the video to measure quality. However, SSIMPLUS goes a step further by considering additional factors, such as viewing conditions and device capabilities, to provide a more personalized measure of quality.SSIMPLUS leverages machine learning and advanced algorithms to provide a highly accurate measure of perceived quality. Unlike traditional metrics, SSIMPLUS does not require a reference video for comparison, making it more flexible and practical for real-world applications.In the industry, SSIMPLUS is widely used for quality control, benchmarking, and optimization purposes. It provides a consistent and repeatable measure of quality, which is essential for comparing different video codecs, streaming protocols, and network conditions. It also provides valuable insights into the viewer's perception of quality, which can be used to optimize video encoding and delivery strategies.
Comparative Analysis
Comparing Metrics: Strengths and Weaknesses
When comparing video quality metrics, it's important to consider their strengths and weaknesses. Each metric has its unique approach and methodology, which may be more or less effective depending on the application.For example, traditional metrics such as PSNR and MSE are simple and easy to compute, making them practical for real-time applications. However, they lack the ability to accurately reflect the viewer's perception of quality, as they do not consider subjective factors.On the other hand, advanced metrics such as AVQT, VMAF, and SSIMPLUS provide a highly accurate measure of perceived quality, as they consider a wide range of factors, including viewing conditions and device capabilities. However, they are computationally intensive and may not be practical for real-time applications.Therefore, the choice of metric should depend on the specific requirements of the application. For real-time applications, a simple and fast metric such as PSNR or MSE may be sufficient. For applications that require a high degree of accuracy, an advanced metric such as AVQT, VMAF, or SSIMPLUS may be more appropriate.
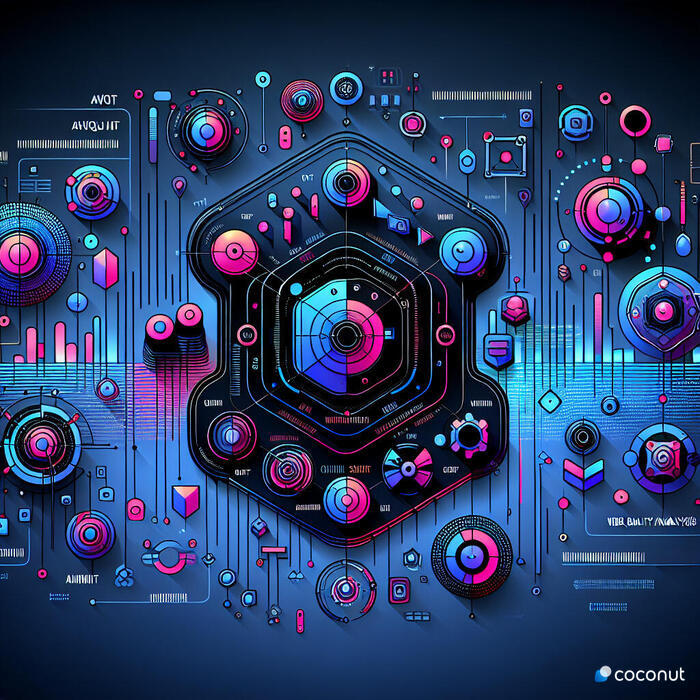
Comparative Analysis: AVQT, VMAF, and SSIMPLUS
In the realm of video quality metrics, AVQT, VMAF, and SSIMPLUS are three prominent players. Each of these metrics has its unique strengths and weaknesses, which we will delve into in this section. AVQT, or Absolute Video Quality Test, is a robust tool that provides a comprehensive assessment of video quality. Its strength lies in its ability to evaluate both the spatial and temporal aspects of video quality, providing a holistic view of the user's viewing experience. However, AVQT's complexity can be a drawback, as it requires significant computational resources and expertise to implement effectively.On the other hand, VMAF, or Video Multi-Method Assessment Fusion, is a metric developed by Netflix that combines several algorithms to evaluate video quality. Its strength lies in its adaptability, as it can be tailored to specific viewing conditions and devices. However, VMAF's reliance on multiple algorithms can also be a weakness, as it may not always provide consistent results across different viewing scenarios.Lastly, SSIMPLUS is a video quality metric that focuses on the perceptual quality of the video. Its strength lies in its ability to accurately predict viewer satisfaction, making it a valuable tool for content providers. However, SSIMPLUS's emphasis on perceptual quality can also be a limitation, as it may not fully capture the technical aspects of video quality.In conclusion, while AVQT, VMAF, and SSIMPLUS each have their strengths and weaknesses, they all play a crucial role in assessing and improving video quality. The choice between these metrics ultimately depends on the specific needs and resources of the content provider.
Metrics in Action: Real-World Applications
To illustrate the practical application and impact of these metrics, let's consider a few real-world examples. A major streaming service, for instance, might use AVQT to evaluate the quality of its content before it is delivered to viewers. By identifying and addressing any quality issues early on, the service can ensure a seamless viewing experience for its users. In another scenario, a content provider might use VMAF to optimize its encoding process. By tailoring the encoding parameters to the specific viewing conditions and devices of its audience, the provider can deliver high-quality content while minimizing bandwidth usage.Lastly, a video platform might use SSIMPLUS to gauge viewer satisfaction. By accurately predicting how viewers perceive the quality of its content, the platform can make informed decisions about its content strategy and delivery methods. These examples underscore the significant impact that video quality metrics can have on content delivery and viewer satisfaction.
Further Readings and Online Resources
- AVQT Compared to VMAF and SSIMPLUS
- Judging Apple's Advanced Video Quality Tool
- VMAF: The Journey Continues
As we've discussed, video quality metrics like AVQT, VMAF, and SSIMPLUS play a pivotal role in content delivery. They provide valuable insights into the quality of video content, enabling content providers to optimize their delivery methods and enhance viewer satisfaction. As technology continues to evolve, we can expect these metrics to become even more sophisticated and integral to the content delivery process.
Before we wrap up, let's touch on the relevance of Coconut in the cloud video transcoding space. Coconut can ensure high-quality video transcoding, contributing to a superior viewing experience for users.