In the digital age, video content has become a dominant force in the world of entertainment, education, and communication. With this surge in video consumption, the importance of video quality assessment has grown exponentially. It's no longer enough to simply create and distribute video content; the quality of that content can significantly impact viewer engagement, satisfaction, and ultimately, the success of the content itself. This is where video quality assessment tools, such as the Structural Similarity Index (SSIM), come into play. SSIM is a method for comparing the similarity between two images or videos. It's a powerful tool that allows content creators, broadcasters, and video streaming services to ensure their content meets the high-quality standards demanded by today's discerning viewers. In this article, we delve into the world of video quality assessment, with a particular focus on SSIM, providing expert insights into its importance, how it works, and why it's a valuable tool in the video content industry.
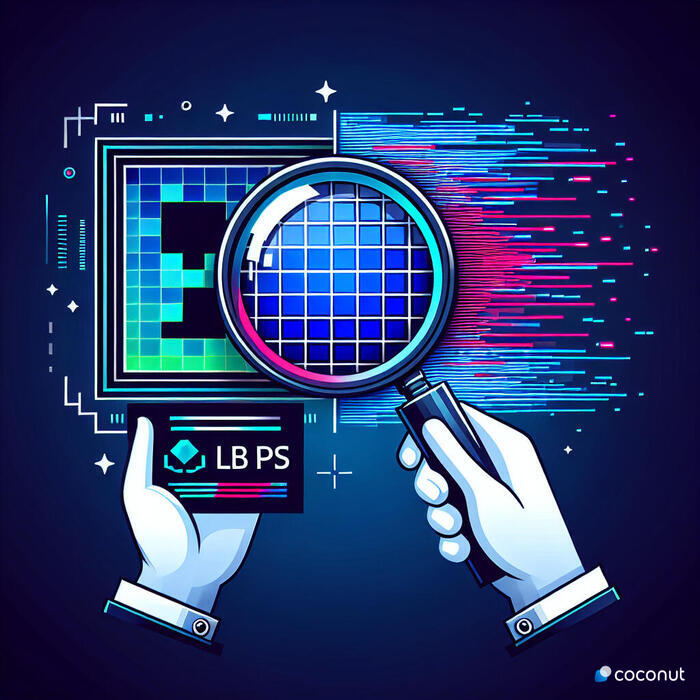
Decoding Video Quality Metrics
Video quality metrics are crucial for anyone involved in the creation, distribution, or streaming of video content. They provide a quantifiable measure of video quality, allowing for objective comparisons and evaluations. These metrics are essential for maintaining high standards of quality, identifying issues, and making necessary improvements. They also play a crucial role in ensuring a positive viewer experience, as poor video quality can lead to viewer dissatisfaction and disengagement. In an industry where viewer retention and engagement are key to success, understanding and utilizing video quality metrics is non-negotiable.
Defining Video Quality
Video quality is a multifaceted concept. From a viewer's perspective, it's about the visual and auditory experience - the clarity of the image, the smoothness of motion, the synchronization of sound and picture. From a technical standpoint, video quality involves various factors such as resolution, bitrate, frame rate, and compression artifacts. It's a delicate balance between maintaining high visual and auditory standards while managing bandwidth and storage constraints.
Exploring Video Quality Metrics
There are several video quality metrics used in the industry, each with its strengths and weaknesses. Peak Signal-to-Noise Ratio (PSNR) is a widely used metric that measures the difference between the original and compressed video. Video Multimethod Assessment Fusion (VMAF) is a metric developed by Netflix that combines several algorithms to predict video quality. Mean Opinion Score (MOS) is a subjective metric based on human evaluations. Each of these metrics provides valuable insights into video quality, but none are perfect. This is where SSIM comes into the picture.
Unveiling SSIM (Structural Similarity Index)
The Structural Similarity Index (SSIM) is a video quality metric that has gained significant attention in recent years. Developed by Zhou Wang, Alan Bovik, Hamid Sheikh, and Eero Simoncelli in 2004, SSIM was designed to improve upon traditional metrics like PSNR by more accurately reflecting perceived visual quality. SSIM operates on the principle that the human visual system is highly adapted to extract structural information from a scene, and therefore, a measure of structural similarity can provide a more accurate reflection of perceived quality.
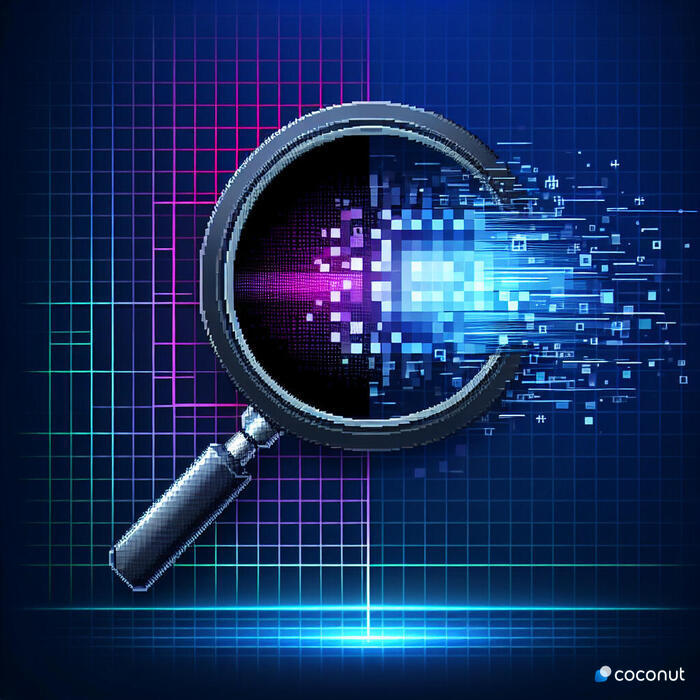
Deciphering SSIM
SSIM is a complex algorithm that compares two images or videos in terms of their luminance, contrast, and structure. The luminance comparison measures the brightness of the images, the contrast comparison measures the dynamic range, and the structure comparison measures the spatial distribution of pixel intensities. The SSIM index is then calculated as a combination of these three comparisons. The beauty of SSIM lies in its ability to mimic the human visual system's ability to perceive quality, making it a powerful tool for video quality assessment.
Appreciating SSIM
The use of SSIM offers several advantages. Firstly, it provides a more accurate reflection of perceived video quality compared to other metrics. This is because it takes into account the structural information that the human visual system is naturally attuned to. Secondly, SSIM is a full-reference metric, meaning it requires access to the original, unprocessed image or video for comparison. This allows for a more accurate assessment of quality as it directly compares the processed video to the original. Lastly, SSIM is relatively easy to compute and interpret, making it a practical choice for real-world applications.
Benefits of Using SSIM for Video Quality Assessment
The Structural Similarity Index Measure (SSIM) is a powerful tool for video quality assessment, offering several key benefits. Firstly, SSIM provides a more accurate representation of perceived video quality. Unlike other metrics that focus on pixel-by-pixel comparisons, SSIM considers changes in structural information, luminance, and contrast, which are more aligned with the human visual system's perception. This results in a more reliable and realistic assessment of video quality. Secondly, SSIM is highly versatile, applicable to a wide range of video content and formats. This makes it a valuable tool for various industries, from broadcasting to video game development. Lastly, SSIM is computationally efficient. It requires less processing power compared to other video quality metrics, making it a cost-effective solution for real-time video quality monitoring. These benefits make SSIM a preferred choice for video quality assessment in many scenarios.
Limitations and Considerations
Despite its advantages, there are certain limitations and considerations when using SSIM for video quality assessment. One of the main limitations is that SSIM may not always correlate perfectly with human visual perception. While it does a better job than many other metrics, there can still be discrepancies, especially in cases of severe distortion or when dealing with high dynamic range content. Additionally, SSIM is not always the best choice for all types of video content. For instance, it may not be as effective for assessing the quality of videos with fast motion or complex textures. Furthermore, while SSIM is computationally efficient, it can still be resource-intensive when applied to high-resolution videos or large video libraries. Therefore, it's crucial to consider these factors when deciding to use SSIM for video quality assessment.
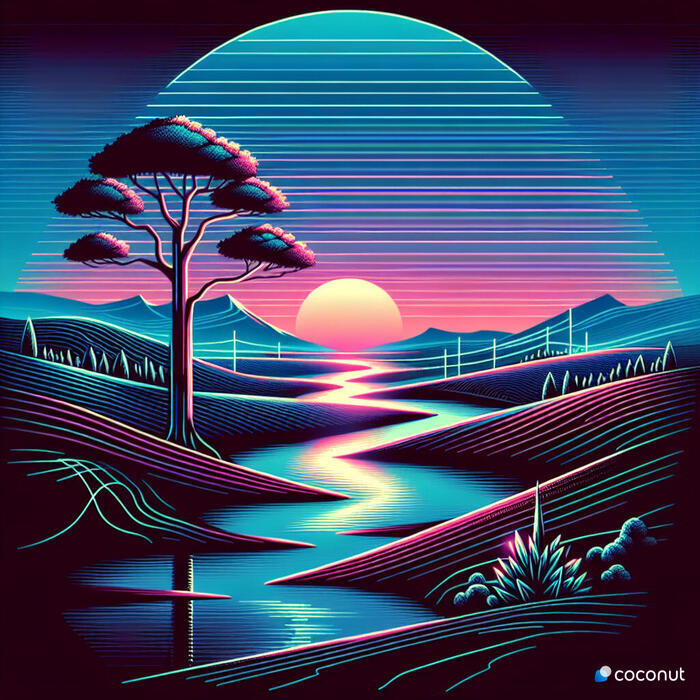
Applying SSIM in Real-World Scenarios
SSIM is widely used in various industries and real-world scenarios for video quality assessment. In the film and television industry, SSIM is used during post-production to ensure the quality of visual effects and color grading. It helps identify any loss of quality that may occur during the editing process, allowing for corrections before the final product is released. In the broadcasting industry, SSIM is used to monitor the quality of live broadcasts. It helps detect any degradation in video quality in real-time, enabling immediate action to prevent poor viewer experience. In the video game industry, SSIM is used during game development to assess the quality of game graphics and animations. It helps ensure that the game visuals meet the desired quality standards, contributing to a better gaming experience. In the field of video compression, SSIM is used to guide compression decisions. It helps balance the trade-off between compression efficiency and video quality, ensuring optimal viewer experience. These are just a few examples of how SSIM is applied in real-world scenarios, demonstrating its versatility and importance in video quality assessment.
SSIM in Content Creation
In the realm of content creation, SSIM plays a crucial role in ensuring the quality of the final product. During post-production, editors often have to manipulate the video in various ways, such as color grading, adding visual effects, or resizing. These manipulations can sometimes lead to a loss of video quality. SSIM can be used to assess the quality of the video after each manipulation, allowing editors to identify and correct any quality degradation. This ensures that the final product maintains the desired level of quality, leading to a better viewer experience. Furthermore, SSIM can also be used to compare different versions of a video, helping content creators choose the version that offers the best quality.
SSIM in Broadcasting and Streaming
SSIM is of paramount importance in the broadcasting and streaming industry. In live broadcasting, maintaining high video quality is crucial for viewer satisfaction. SSIM can be used to monitor the quality of the broadcast in real-time, allowing for immediate action if any degradation is detected. This helps prevent poor viewer experience due to low-quality broadcasts. In streaming services, SSIM can be used to assess the quality of the video after compression. This helps ensure that the video maintains a high level of quality even after being compressed for streaming, leading to a better viewer experience. Furthermore, SSIM can also be used to compare the quality of different streams, helping service providers choose the stream that offers the best quality.
SSIM in Video Compression
SSIM plays a significant role in guiding video compression decisions. Video compression is a trade-off between reducing the file size and maintaining video quality. SSIM can be used to assess the quality of the video after compression, providing a quantitative measure of how much the quality has been degraded. This information can be used to adjust the compression parameters, balancing the need for efficiency with the desire for high video quality. This ensures that the compressed video maintains a high level of quality, leading to a better viewer experience. Furthermore, SSIM can also be used to compare the quality of different compression algorithms, helping choose the algorithm that offers the best balance between compression efficiency and video quality.
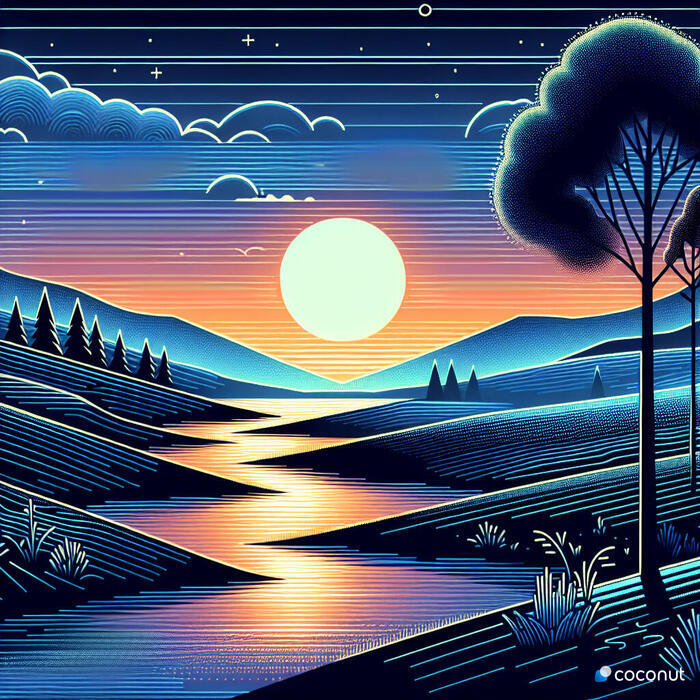
Comparing SSIM with Other Video Quality Metrics
SSIM is often compared with other video quality metrics to understand its strengths and weaknesses. One such metric is the Peak Signal-to-Noise Ratio (PSNR), which is a simple and widely used metric for video quality assessment. However, PSNR focuses on pixel-by-pixel comparisons and does not consider changes in structural information, luminance, and contrast. This can lead to discrepancies between the PSNR score and the perceived video quality. On the other hand, SSIM considers these factors, providing a more accurate representation of perceived video quality. Another metric often compared with SSIM is the Video Multimethod Assessment Fusion (VMAF), developed by Netflix. VMAF combines several video quality metrics, including SSIM, to provide a comprehensive assessment of video quality. While VMAF can provide a more detailed assessment, it is also more complex and computationally intensive than SSIM. Therefore, the choice between SSIM, PSNR, and VMAF depends on the specific requirements of the video quality assessment task.
SSIM vs. PSNR
When comparing SSIM with PSNR, there are several key differences to consider. PSNR is a simple and straightforward metric that compares the original and distorted videos on a pixel-by-pixel basis. However, this approach does not always correlate well with human visual perception, as it does not consider changes in structural information, luminance, and contrast. On the other hand, SSIM considers these factors, providing a more accurate representation of perceived video quality. Furthermore, SSIM is more versatile and applicable to a wider range of video content and formats. However, PSNR is less computationally intensive than SSIM, making it a more suitable choice for scenarios where computational resources are limited. Therefore, the choice between SSIM and PSNR depends on the specific requirements of the video quality assessment task.
SSIM vs. VMAF
Comparing SSIM with VMAF, each has its strengths and weaknesses. VMAF is a comprehensive video quality metric that combines several other metrics, including SSIM. This allows VMAF to provide a more detailed and accurate assessment of video quality. However, VMAF is also more complex and computationally intensive than SSIM. On the other hand, SSIM is a simpler and more efficient metric that still provides a reliable assessment of video quality. Furthermore, SSIM is more versatile and applicable to a wider range of video content and formats. However, VMAF may provide a more accurate assessment in certain scenarios, such as when dealing with high dynamic range content or complex textures. Therefore, the choice between SSIM and VMAF depends on the specific requirements of the video quality assessment task.
Advancements and Future of Video Quality Assessment
The field of video quality assessment is continuously evolving, with new advancements and technologies emerging regularly. One of the key trends is the increasing use of machine learning and artificial intelligence in video quality assessment. These technologies can be used to develop more sophisticated and accurate video quality metrics, potentially surpassing traditional metrics like SSIM, PSNR, and VMAF. Furthermore, there is a growing focus on developing video quality metrics that can accurately assess the quality of immersive video content, such as virtual reality and 360-degree videos. These types of content present unique challenges for video quality assessment, requiring new approaches and metrics. As for SSIM, it will continue to be a valuable tool for video quality assessment, thanks to its reliability, versatility, and efficiency. However, it will also need to evolve and adapt to the changing landscape of video content and technologies.
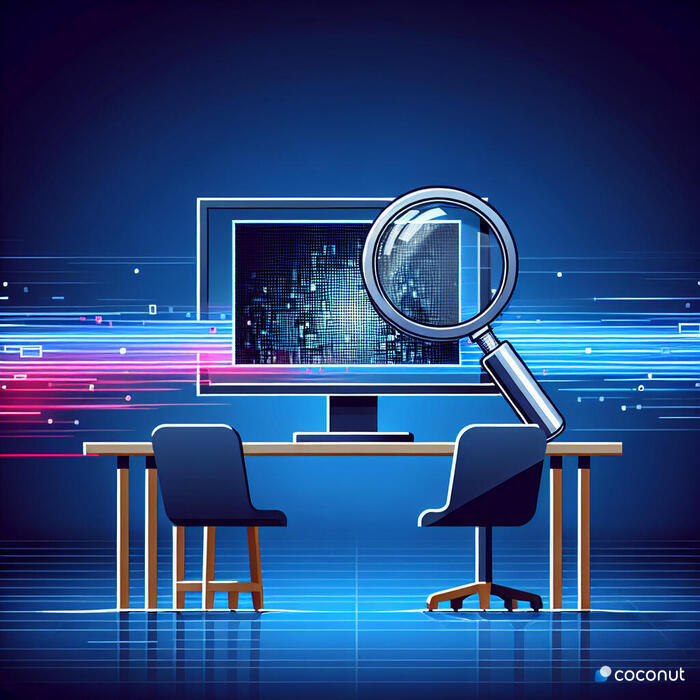
Video Quality Assessment: The Evolution of SSIM and Beyond
In the realm of video quality assessment, the Structural Similarity Index (SSIM) has been a game-changer. It has revolutionized the way we perceive and measure video quality, offering a more accurate and comprehensive evaluation than traditional methods. As we delve into the latest advancements in video quality assessment, we find that SSIM continues to be a pivotal metric, but it's not the only player in the game. The landscape is evolving, with new technologies and methodologies emerging, and the influence of machine learning and artificial intelligence (AI) becoming more pronounced. In this article, we'll explore these developments and discuss the future direction of metrics like SSIM.
Emerging Technologies in Video Quality
The field of video quality assessment is in a constant state of flux, with new technologies and methodologies emerging at a rapid pace. One such advancement is the use of deep learning algorithms for video quality prediction. These algorithms can learn from large amounts of data and make accurate predictions about video quality, even in complex and dynamic scenarios. Another emerging technology is the use of cloud-based video quality assessment tools. These tools offer scalability and flexibility, allowing for real-time video quality assessment across multiple devices and platforms. Furthermore, the advent of 4K and 8K video formats has necessitated the development of new metrics and methodologies to accurately assess the quality of these high-resolution videos. These advancements are pushing the boundaries of what's possible in video quality assessment, paving the way for more accurate and comprehensive evaluations.
The Role of Machine Learning and AI
Machine learning and AI are playing an increasingly important role in the development of video quality metrics. These technologies are capable of analyzing vast amounts of data and identifying patterns that humans might miss, leading to more accurate and nuanced assessments of video quality. For instance, machine learning algorithms can be trained to recognize and quantify visual artifacts such as blurring, blocking, and banding, which are common issues in compressed videos. AI, on the other hand, can be used to develop predictive models that can anticipate the perceived quality of a video based on various factors, such as the encoding parameters and network conditions. These technologies are not only enhancing the accuracy of video quality metrics but also making the assessment process more efficient and scalable.
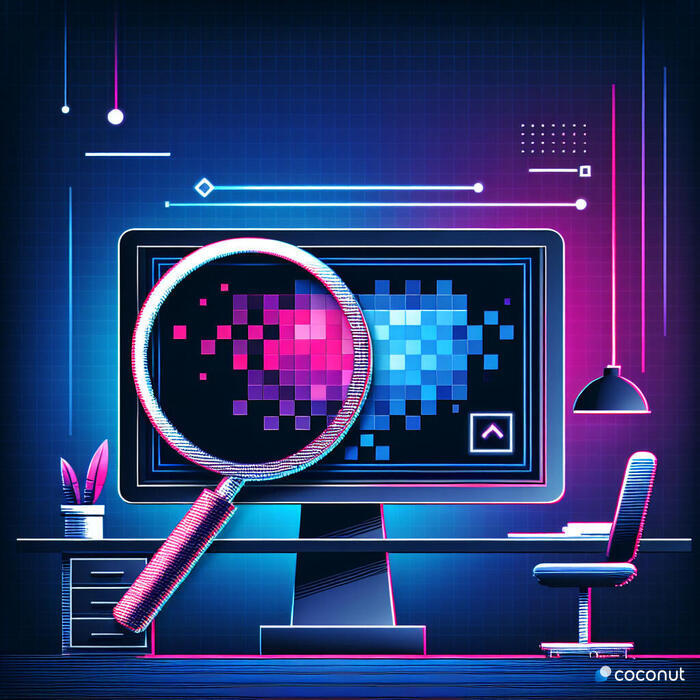
The landscape of video quality assessment is evolving rapidly, with new technologies and methodologies emerging and the influence of machine learning and AI becoming more pronounced. SSIM, while still a pivotal metric, is no longer the only player in the game. The advent of deep learning algorithms and cloud-based assessment tools, coupled with the rise of high-resolution video formats, is pushing the boundaries of what's possible in video quality assessment. However, despite these advancements, the goal remains the same: to accurately and comprehensively evaluate the perceived quality of a video. In this regard, SSIM continues to be a valuable tool, offering a robust and reliable measure of structural similarity between a reference video and a distorted video. But as the field continues to evolve, we can expect to see new metrics and methodologies that build upon the foundations laid by SSIM, offering even more accurate and nuanced assessments of video quality. The future of video quality assessment is exciting and full of potential, and we look forward to seeing what it holds.